As consultants, we’ve worked with hundreds of organizations across industries to help them design the future, which, of course, includes how they think about AI.
The narrative around generative AI tends to be through a big-picture, ideological lens, but in the near future, every industry will have the opportunity to incorporate it. Imagine a world where:
- On-demand access to healthcare diagnoses, medical advice, and monitoring is widely available.
- Tailored education meets every student where they are.
- Systems manage renewable energy and help us tailor use to save costs and preserve our natural resources.
- Banks and financial systems guide us on our financial journey and goals no matter our level of financial literacy.
- Government employees focus on orchestrating policy and supporting citizens instead of drowning in data and documents.
AI has huge promise. And if done well, it can make huge improvements. So what can we do today? How can we take advantage of this superpower while managing the inherent risks? Let’s start with how we got here.
The birth of superpowers
Companies have used machine learning (ML) for years for its behind-the-scenes automation capabilities. Then came the boom of large language models (LLMs) that allowed computers to understand natural language and human intent (enter: customer service chatbots).
The more recent onset of Gen AI made machine learning possible. Gen AI is powerful, and the considerations for leveraging it properly and responsibly are growing increasingly complex.
Related: Design Responsibly To Earn Trust
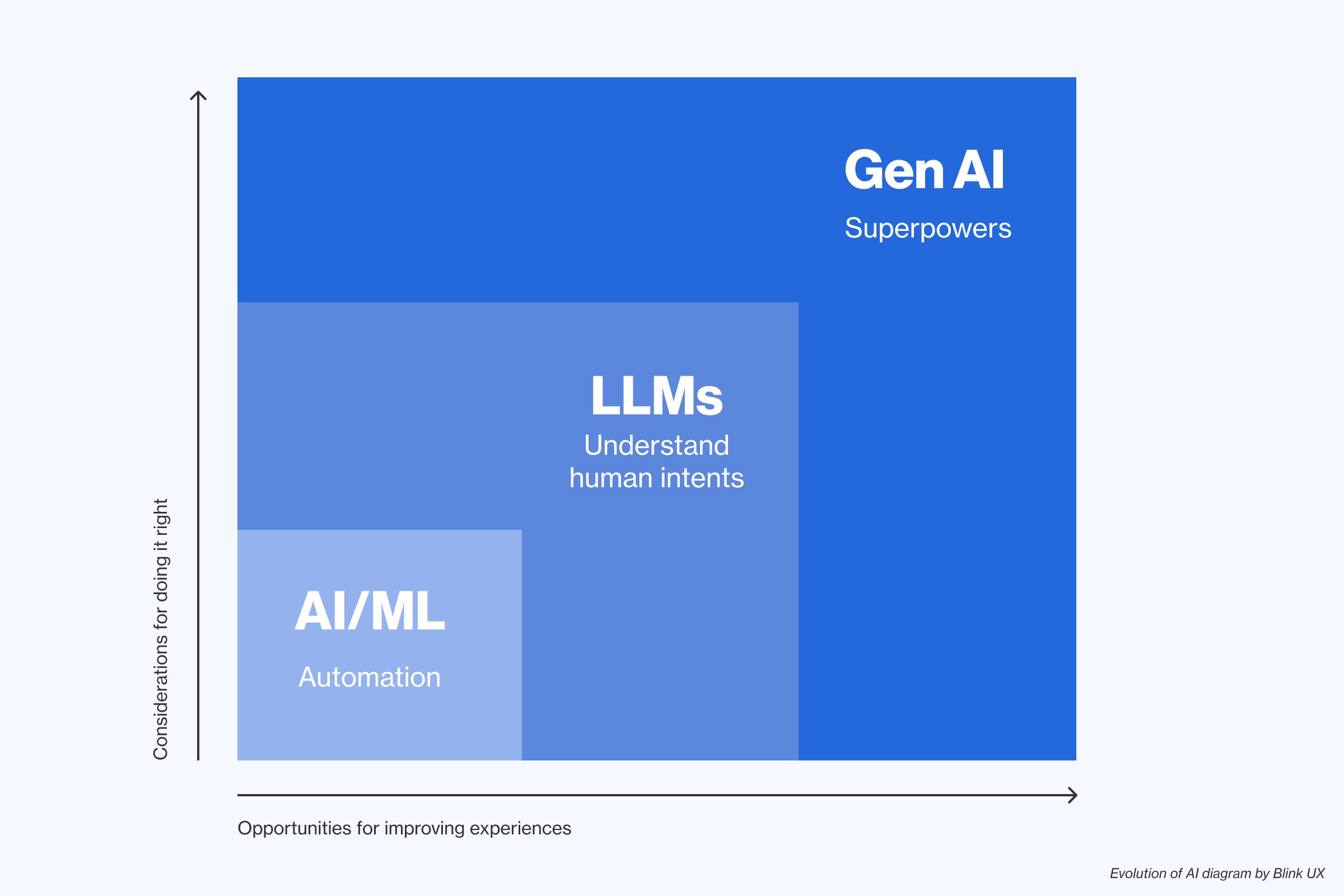
Opportunities and considerations
We believe the most successful companies think about both the customer and the employee — those who deliver the customer experience — simultaneously.
With the customer experience, AI gives businesses the opportunity to deliver more innovative and accessible products and solutions, but not without significant considerations around privacy. Customers want to know what you’re doing with their data — transparency of what’s hidden behind that black box and the ethics to avoid exclusion.
On the internal side, there’s an opportunity to help employees work better and more efficiently, focusing on the most valuable functions of their jobs instead of the less impactful, repetitive, and time-consuming tasks. But implementing AI internally comes with a wealth of considerations, like security and confidentiality of intellectual property, protecting customer privacy, and ensuring the technology works as intended (a mistake in Netflix’s recommendation algorithm is far less harmful than automation gone wrong in a power plant or hospital administration software). Attending to and establishing the proper level of human control is also essential.
What you can do now: A four-step framework for adopting AI
This four-step framework outlines how to take advantage of AI while managing the risks of misinformation, data privacy, hallucination, and inaccuracy.
1. Start with the people, not the technology.
Start by gaining a deeper understanding of your customer journeys and employee workflows to identify the pain points and opportunities for improvement. In this stage, focus solely on identifying pain points and areas for improvement before you think about ways AI can help. Once you have a complete understanding of what your users want and need, you can identify where AI fits in.
Case study: Edelman Financial Engines (EFE)
EFE is looking at creating the perfect balance between the power of algorithmic investing and the human touch customers crave. Through extensive customer research, EFE learned that a customer’s relationship with their advisor is paramount, and informing customers how and when they use AI will significantly boost trust. As a result, EFE is investing in ways to automatically highlight how they’re using AI while helping advisors focus less on busy work and more on big-picture value to help customers reach their financial goals.
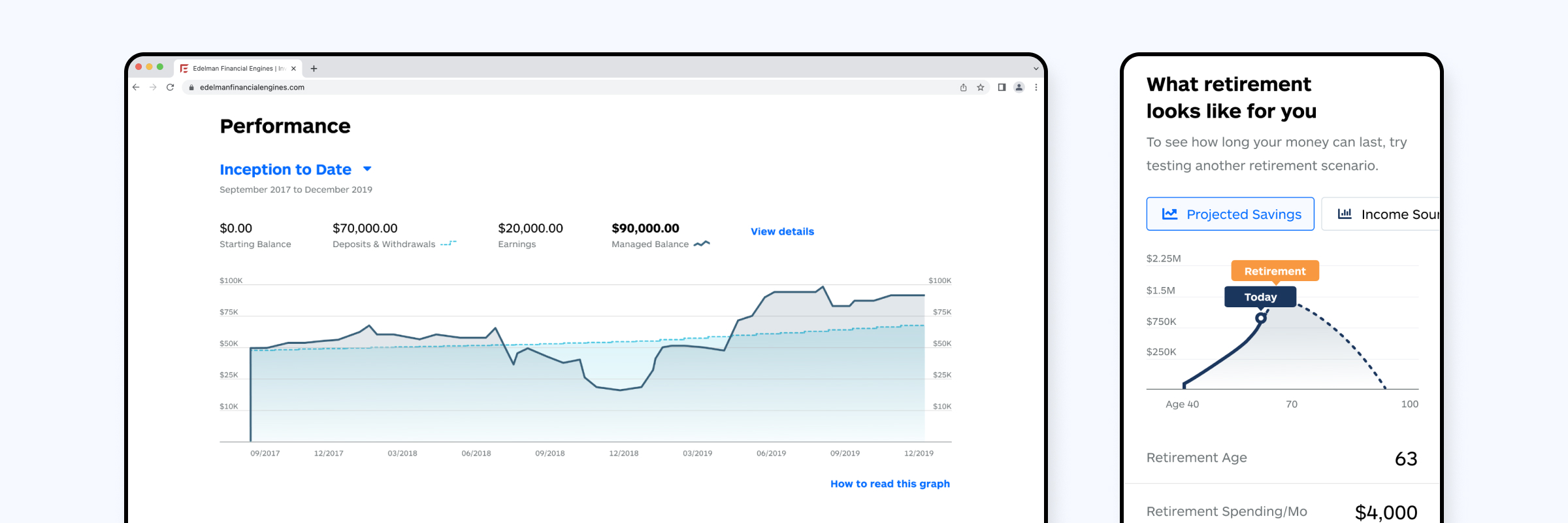
2. Zero in on opportunities and think big
Once you’ve uncovered the pain points and opportunities, it’s time to go big and broad on generating ideas for providing value and solving your users’ pain points. This is a great time to think about how you want to apply AI-enabled solutions, build user trust, and provide the right level of transparency and human control.
Case study: Duolingo
Duolingo is a top app for learning new languages and one of my favorite examples for finding opportunities to automate. They asked themselves, “How can AI help learners in a way that’s unique to our brand?” and recently launched Duolingo Max with features like AI-powered language tutors. They uncovered their users's pain points (learning new languages) and found an opportunity to leverage AI in a way that’s unique and authentic to their brand. They’re also working with language experts to make sure they remain authentic and get it right.
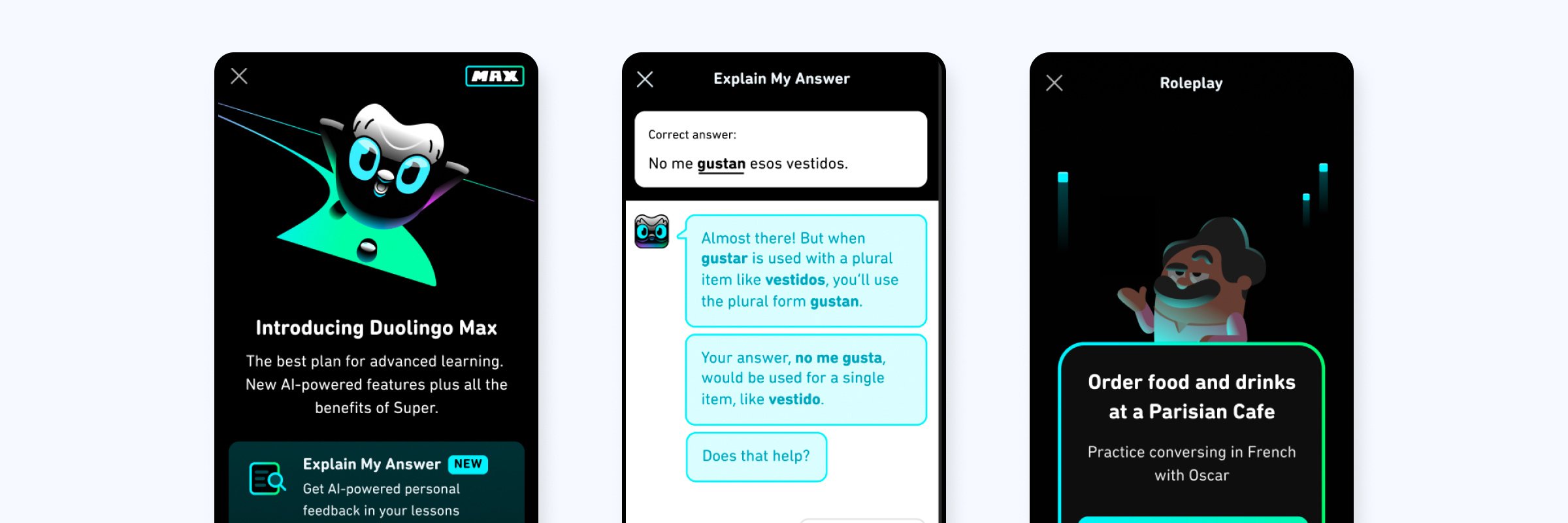
3. Train your model ethically, inclusively, and accurately
An AI model is only as good as the data it receives, and all generative AI models are prone to error and bias. Take the time — especially with proprietary data — to think through the necessary training.
Case study: Adobe
Adobe recently released its AI-based image-generating software Firefly, and behind the scenes, they’re being very intentional about how it works. They know there are limitations when it comes to inherent bias; representation of different cultures, genders, races; and hallucinations. They’ve set up an inclusive and responsible design group whose job is to determine where errors and misrepresentations are present to retrain the software for inclusivity.
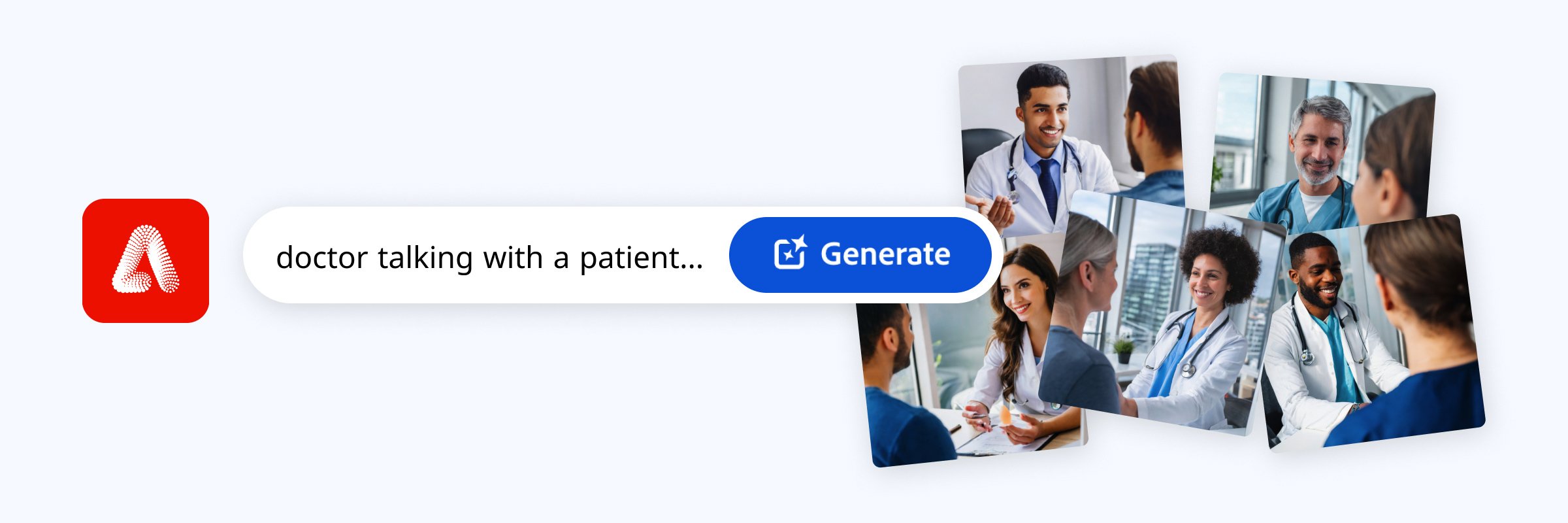
4. Monitor and improve
An AI solution is never flawless on day one. Establish a practice of continually monitoring and using insights to drive improvements. One great way to do this accurately and efficiently across different business units is to create a unified system to inform and track every type of research you conduct (i.e., quantitative, qualitative, customer research, AB testing). Develop a framework that consolidates your findings to evaluate how well you meet your customer or employee improvement goals. From there, make the necessary adjustments.
Case study: Utilities Industry
One of our major utility clients is leveraging machine learning to reroute power during outages faster than humans to ensure customers avoid costly losses. Their dedication to constantly monitoring and enhancing their automated outage system ensures their use of AI provides the best value to customers.
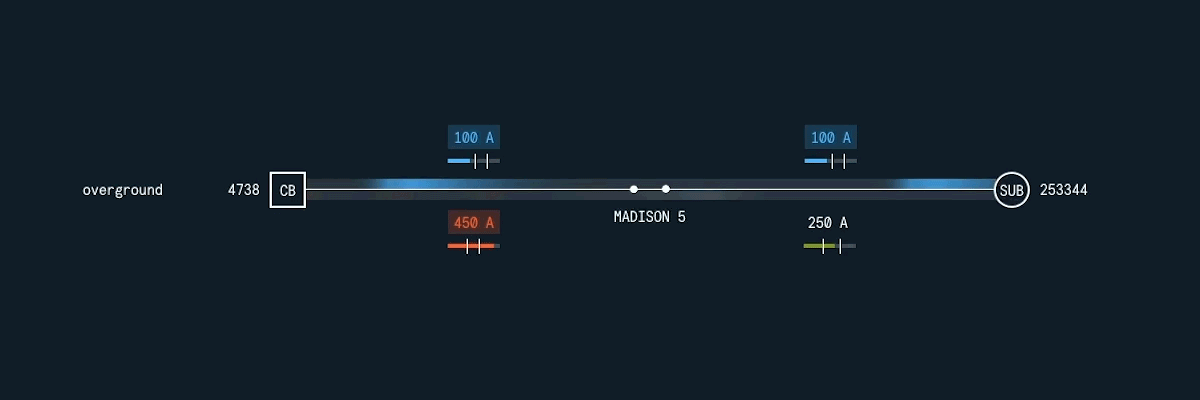
Better AI for a better tomorrow
With the explosion of Gen AI and LLMs, there’s great potential to make some of the previously impossible possible. So, how can we get there in an ethical way? How can AI better our healthcare, government, and education systems?
At Blink, we focus on uncovering the highest value experiences on every engagement, some of which require AI solutions. Through our evidence-driven approach, we learn from users, explore widely, create concepts that meet human needs, and recommend which of the most successful concepts would be powered by AI and how to track their success. We’re also developing unique approaches within our evidence-driven design process to specifically explore how AI can benefit our clients' products, customers, and businesses.
Ready to transform your customer and employee experiences with AI? We’ll help you get started. Share your goals, and let’s create an evidence-driven solution.